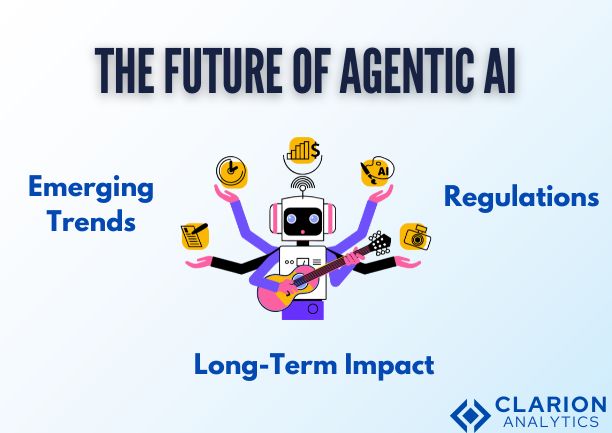
1. Introduction
-
Hook: The rise in Agentic AI in the landscape of AI systems has given birth to intelligent systems which have a certain level of autonomy which to a degree is comparable to a Human .
-
Definition: Agentic AI are intelligent systems which have the ability to perceive the environment , which helps them enhance their capabilities to perform a given set of tasks more accurately , efficiently and has a higher level of autonomy when compared to traditional AI systems.
-
Purpose: The purpose of this blog is to grasp the structure of Agentic and elucidate its significance in the ongoing development of artificial intelligence
2. What is Agentic AI?
-
Definition: Agentic AI is a branch of AI systems which emphasizes Autonomy , Goal-oriented Behavior and environment adaptability for better decision making , in contrast with the traditional AI systems which operate within fixed parameters range and lack the ability to adapt and learn from the environment.
-
Examples: Real-world applications are increasingly prevalent across various sectors. For instance, autonomous vehicles utilize sophisticated sensors and algorithms to navigate and make real-time decisions based on environmental data, thereby enhancing transportation safety and efficiency.
-
Significance: Understanding it is essential for ethical, technical, and practical reasons. Ethically, it highlights the need to balance autonomy with human oversight, as these systems can independently make impactful decisions. Technically, it equips developers to build adaptive and reliable AI that meets evolving needs.
3. The Key Components of Agentic AI
a. Perception:
Agentic AI systems obtain their environmental understanding through multiple sensors in conjunction with data processing operations. Autonomous vehicles utilize LiDAR together with cameras and radar to obtain environmental data that their computer vision algorithms translate into safe obstacle identification and route navigation
b. Decision-Making:
The combination of reinforcement learning and game theory algorithms helps decision-making processes. These computing systems allow evaluation of possible actions through their predicted outcomes resulting in decisions that fulfill designated targets and operational boundaries
c. Goal Formulation:
Agentic Identifies priorities together with objectives then sets explicit goals toward them through a series of reasoning and action processes which generate results for complex tasks and queries through goal formulation.
d. Action Execution:
Systems need to execute decisions through their actions following the completion of selection processes in dynamic operational spaces. Both robotic physical operations such as warehouse navigation and software trading in financial markets comprise elements of goal-based execution.
e. Feedback Loops:
Continuous learning and adaptation are facilitated by feedback loops in Agentic AI systems
4. Architectures Supporting Agentic AI
a. Hierarchical Models:
Agentic AI systems implement hierarchical decision systems as they tackle diverse complex operational tasks. The control system of autonomous vehicles implements different layers of decision protocols that translate strategic plans into actionable real-time operations.
b. Hybrid Architectures:
Engineers implement hybrid architectural solutions that blend symbolic processing with machine learning to deliver improved functionality. Healthcare cognitive AI solutions merge medical rules with AI learning capabilities to generate individualized treatment suggestions.
c. Multi-Agent Systems:
A Multi-Agent System (MAS) uses autonomous agents to work together for reaching intricate targets through joint efforts. The concept behind swarm robotics demonstrates how multiple robots collaborate through this approach during search and rescue operations as well as supply chain optimization tasks.
5. Challenges in Designing Agentic AI
a. Ethical Concerns:
The development of it generates major ethics problems between human control and autonomy. Additional decision-making autonomy in systems creates multiple issues regarding responsible accountability and ethical conduct analysis.
b. Technical Challenges:
The present algorithms and hardware systems encounter restrictions which limit the performance of Agentic AI. The field continues to face limitations related to how data from restricted sources will be generalized and performance limitations that affect scalability alongside computational efficiency problems.
c. Security Risks:
Security risks affect systems because they contain weaknesses that make them vulnerable to adversarial attacks targeting their decision-making procedures. Adequate security measures must be implemented to both protect from harmful use and provide safe operation of these technologies.
6. Real-World Applications of Agentic AI
a. Autonomous Vehicles represent adaptive driverless transportation that performs safe and efficient operations independently of human operating conditions.
b. The process of industrial automation combines manufacturing workflow optimization through machine-operated complex task completion after breaking down large problems into smaller manageable ones to solve them collaboratively for complex output.
The implementation of AI systems continues to advance healthcare operations because they offer personalized medical diagnosis insight and treatment planning routes which improve both patient care and therapeutic outcomes.
d. Agentic systems use cameras for smart city management to enhance energy systems and traffic control and public safety operations.
7. The Future of Agentic AI
Self-improving AI systems together with advanced decision-making models function as emerging trends that define upcoming technology developments. New developments ensure better capabilities and more applications for AI systems in various domains.
Agentic AI-based changes in society could bring large-scale transformations which would affect both economic systems and social structures as well as healthcare systems and urban development.
Protecting society from future technology requires responsible policies for governing emerging systems as their capabilities continue to grow. Protecting public good will require proper ethical frameworks and regulatory structures to guide safe system deployment that brings benefits to society.
8. Conclusion
- In summary, understanding the structure of Agentic AI is critical for harnessing its potential while addressing the ethical, technical, and practical challenges it presents. As these systems become more integrated into various aspects of life, a comprehensive understanding of their capabilities and limitations will be essential for fostering responsible development and deployment.
- Are you intrigued by the possibilities of AI? Let’s chat! We’d love to answer your questions and show you how AI can transform your industry. Contact Us