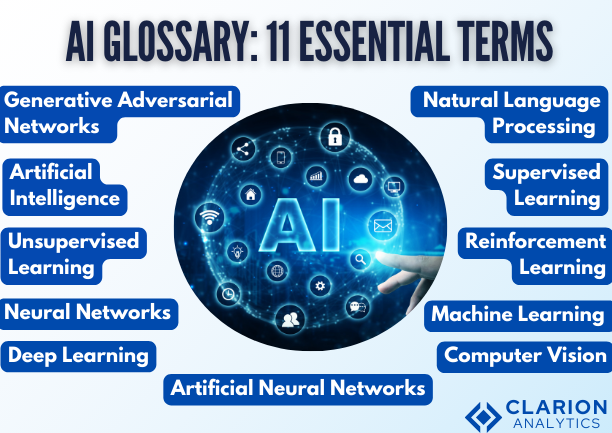
Artificial Intelligence is an ever-evolving field. To both beginners and experts, understanding the terms is important. From foundational concepts to advanced techniques, knowing these terms will help you navigate discussions with confidence. Whether you are a business leader, a software developer, or simply curious about Artificial Intelligence, this Artificial Intelligence glossary clearly explains 11 essential terms. These are industry-standard keywords and play a critical role in understanding the fundamental aspects of Artificial Intelligence.
Our extensive Artificial Intelligence glossary offers detailed definitions of 11 essential terms commonly used throughout the industry. These terms are fundamental elements and are a must-read for anyone who wants to further their knowledge about this fast-evolving field. By getting to know these fundamental concepts, you’ll be able to better relate to Artificial Intelligence subjects and harness its potential better in your work or personal life.
1. Artificial Intelligence (Ai)
Definition:
Artificial Intelligence refers to the imitation of human intelligence in machines that are programmed to execute tasks that normally need human intelligence, like visual perception, speech recognition, decision-making, and language translation.
Significance in AI:
Artificial Intelligence is the general term that covers a range of technologies such as machine learning, natural language processing, and robotics. It is the central idea behind the digital revolution, revolutionizing industries from healthcare to finance.
Application in Real Life:
Artificial Intelligence that fuels virtual assistants such as Siri and Alexa fuels recommendation software on platforms like Netflix, and even assists fraud detection in financial systems.
2. Machine Learning (ML)
Definition:
Machine Learning is one of the subset branches of Artificial Intelligence that facilitates systems to learn from data, detect patterns, and make choices with little to no human interaction.
How It Works:
ML algorithms apply statistical techniques to “train” models on large sets of data, enabling them to learn and perform better over time without being specially programmed for individual tasks.
Real-World Application:
ML has numerous applications in predictive analytics, including predicting stock prices, suggesting products in e-commerce, and spam detection in emails.
3. Deep Learning (DL)
Definition:
Deep Learning is a branch of machine learning that replicates the way the human brain processes information and develops patterns to make decisions. It includes many-layered neural networks (“deep”).
Key Features:
Deep learning is good at dealing with massive amounts of unstructured data, such as images, sound, and text, by employing frameworks like Convolutional Neural Networks (CNNs) and Recurrent Neural Networks (RNNs).
Real-World Application:
DL is responsible for image recognition, autonomous vehicles, and real-time translation.
4. Natural Language Processing (NLP)
Definition:
Natural Language Processing allows computers to read, comprehend, and produce human language. It narrows the space between human communication and machine comprehension.
Key Aspects:
NLP entails activities such as sentiment analysis, language translation, and chatbot development, leveraging tokenization, stemming, and named entity recognition techniques.
Real-World Application:
Common uses are voice-controlled assistants, real-time translation, and content analysis.
5. Neural Networks
Definition:
Neural Networks are a chain of algorithms that simulate the operation of the human brain, especially when it comes to pattern recognition and decision-making.
Principal Structure:
A neural network is composed of layers of interconnected nodes (neurons), each with specific weights and biases, which are optimized through training.
Practical Application:
Neural networks form the basis of deep learning, driving such applications as handwriting recognition, speech synthesis, and even generating works of art.
6. Supervised Learning
Definition:
Supervised Learning is a method of machine learning in which models are trained using labeled data–data containing both input and the correct output.
How It Works:
The model learns from this data to predict outcomes on unseen data. For example, given images labeled as ‘cat’ or ‘dog’, the model learns to classify new images accurately.
Real-World Application:
Supervised learning is used in email spam detection, medical image classification, and customer sentiment analysis.
7. Unsupervised Learning
Definition:
Unsupervised Learning is a form of machine learning wherein the algorithm receives data that has not been labeled or classified. The system attempts to learn about the data’s structure in order to find patterns or groupings.
Key Techniques:
Some typical unsupervised learning methods include clustering (such as k-means) and dimensionality reduction (such as PCA).
Real-World Application:
Unsupervised learning has applications in customer segmentation, outlier detection, and data visualization.
8. Reinforcement Learning (RL)
Definition:
Reinforcement Learning is a form of machine learning in which an agent learns to make decisions by taking actions in an environment to achieve a maximum reward.
Key Elements:
Reinforcement learning includes agents, environments, states, actions, and rewards. The agent learns by trial and error, which makes it suitable for dynamic decision-making environments.
Real-World Application:
RL is applied in game Artificial Intelligence, robotics, and autonomous vehicles.
9. Artificial Neural Networks (ANNs)
Definition:
Artificial Neural Networks are computer models that are based on the neural structure of the human brain and are used for pattern recognition and tasks such as classification and prediction.
Key Features:
ANNs have input, hidden, and output layers. Every neuron receives the input signals and sends them through an activation function that determines the end output.
Real-World Application:
ANNs are used in applications such as facial recognition, predictive maintenance for manufacturing, and medical diagnosis.
10. Generative Adversarial Networks (GANs)
Definition:
Generative Adversarial Networks are a type of machine learning architecture consisting of two neural networks, a discriminator, and a generator, which compete with each other to produce synthetic data that is as real as possible.
How It Works:
The generator produces artificial data and the discriminator checks the data. The generator gets better until the discriminator is not able to differentiate between real and artificial data.
Real-World Application:
GANs are applied in generating realistic images, and videos, and even synthetic data for training .
11. Computer Vision (CV)
Definition:
Computer Vision is an area of Artificial Intelligence aimed at allowing machines to read and comprehend visual information from the world around them.
Key Aspects:
Computer Vision encompasses activities such as object detection, image classification, and image segmentation, typically through deep learning methods.
Real-World Application:
CV is applied in autonomous vehicles, security monitoring, medical diagnostics, and commerce (e.g., visual search).
Conclusion
Understanding these 11 key terms is important for anyone who is involved in the technology field, whether you’re working with AI directly or dealing with AI experts. Understanding basic concepts like machine learning and neural networks, and more advanced concepts like GANs and reinforcement learning, will allow you to move through the AI landscape with more confidence and understanding. It is essential to this knowledge to remain competitive in the changing landscape of AI and to be current with the newest developments.
Intrigued by the possibilities of AI? Let’s chat! We’d love to answer your questions and show you how AI can transform your industry. Contact Us