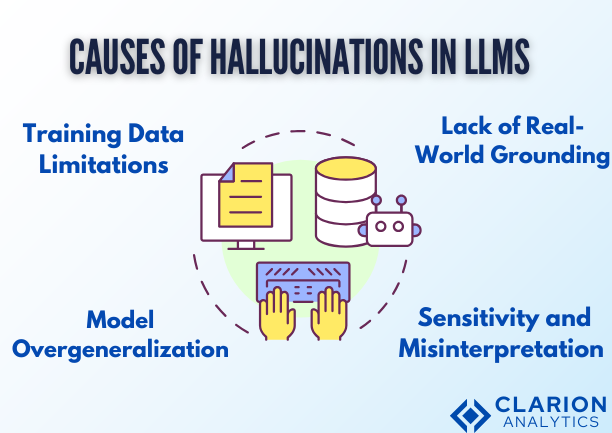
1. Understanding LLM Hallucinations and Their Risks
When I initially started studying huge language models, I was fascinated by their capacity to create cohesive, human-like prose. But, over time, I’ve realised that this very skill contains a terrible flaw: LLM hallucinations. To me, hallucination is more than a technological problem; it is a fundamental restriction of how these models handle information. Consider reading a well-written article that references non-existent research or misinterprets historical facts. This is what many LLM Hallucination do.
One occurrence that stuck with me included an AI-generated legal document referring to a hypothetical court case. It seemed sleek and professional, yet the specifics were completely imagined. This is not only an intellectual issue. In healthcare, for example, I’ve seen models recommend therapies based on out-of-date recommendations, endangering patient safety. Similarly, in finance, hallucinated market projections can cause investors to make disastrous judgements.
What disturbs me the most is how easily these flaws blend into otherwise respectable results, making them difficult to identify. MarkTechPost found that even advanced models such as GPT-4 struggle with factual foundation. This is not surprising. LLMs are taught to anticipate the next word, not to determine the truth. For me, the message is clear: naively believing AI-generated material is risky. We require a culture shift in which consumers treat LLM outcomes with mistrust and double-check key statements. Only then can we reduce risks such as disinformation, reputational loss, and legal responsibility.
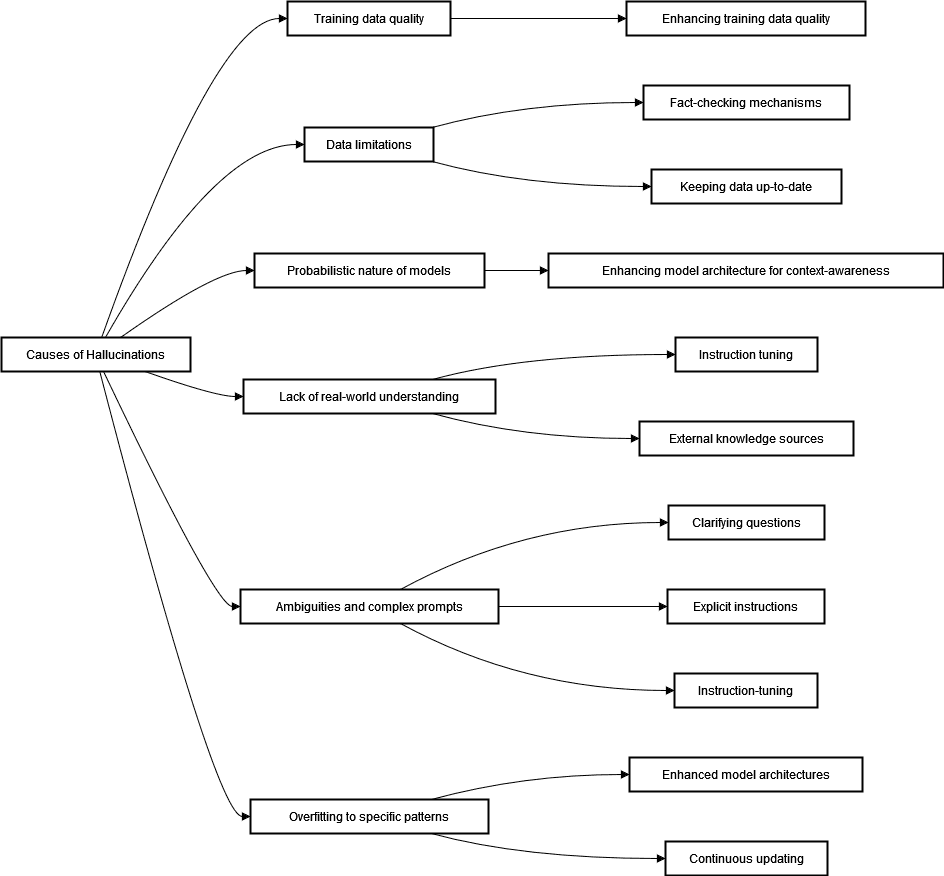
2. Causes of Hallucinations in LLMs
Why are these models hallucinating? From my opinion, the main reasons are inherent in their design. Let’s start with the training data. LLM Hallucination process terabytes of text, but the data is skewed with biases, inconsistencies, and mistakes. I once examined a dataset used to train a medical AI and discovered items from unverified forums alongside peer-reviewed articles. Even people struggle to differentiate reputable sources from noise, so how can a model?
Another consideration is the statistical character of LLM Hallucination. They do not “understand” context; rather, they replicate patterns. For example, if a model sees that the phrase “Einstein invented X” appears frequently, it may generate “Einstein invented quantum computing”—which is untrue. This overgeneralization, as explained in a Springer research results from a failure to understand causation..
Additionally, I’ve seen that cues are essential. While a specific request such as “Summaries the 2023 IPCC report” lessens LLM hallucinations, a generic one like “Explain climate change” may produce a mixture of facts and falsehoods. The mitigation technique of rapid engineering is emphasized by tools such as Haptik’s hallucination detection framework . However, models lack a real-world foundation, even with ideal cues. They are unable to stop in the middle of a statement and question, “Does this make sense?” unlike humans.
3. Prevention Strategies to Reduce Hallucinations
So, how can we resolve this? A multi-layered approach is suggested by my readings and experiments. First, there is no compromise on data quality. Training datasets need to be thoroughly screened to eliminate anything that is out-of-date, biased, or unverified. It’s like tutoring a student: trash in, trash out. In a Springer article, the case is made for domain-specific curation, in which datasets are customized for sectors such as law or healthcare. Next, my preferred method is retrieval-augmented generation (RAG).
RAG helps models to bridge the gap between accuracy and creativity by allowing them to access real-time data from reliable databases. One example of how integrating LLM Hallucination with validated knowledge bases reduces hallucination rates is the blog of SingleStore
But technology isn’t sufficient on its own. Human-in-the-loop systems are something I strongly support. Models may learn from corrections using reinforcement learning from human feedback (RLHF), much like a writer might when they revise their work in response to editor comments. The case study from Quantzig shows how RLHF decreased hallucinations in customer support chatbots by 40%.
And last, openness is important. Users have a right to know when an AI is unsure. By using confidence scores, such as “This answer is 80% reliable based on X sources,” well-informed judgements may be made possible. Developers can benefit from technological protections provided by tools such as Baeldung’s hallucination detection method
4. Future Directions and Ethical Considerations
Looking ahead, I’m divided between being optimistic and cautious. Technologically, I’m interested about advances such as neuromyotonic AI, which combines neural networks with symbolic thinking. Consider an LLM Hallucination that cross-references each claim with a knowledge graph; this may revolutionize accuracy. A paper on arXiv investigates analogous systems, and I’d want to see real-world instances.
However, there are some serious ethical considerations. Who is responsible if a patient is hurt as a result of hallucinated medical advice? How can we achieve a balance between innovation and accountability? My conversations with AI ethicists convinced me that regulation is necessary. Governments must demand openness, such as the EU’s AI Act, which requires the publication of AI-generated data. However, regulation alone will not enough.
We need a culture shift in which developers value safety before speed. Making mitigation tools open source and encouraging cross-industry collaboration are very essential to me. After all, the goal isn’t to eliminate AI; rather, it’s to ensure that it serves people correctly. As I often say in my presentations, “LLM Hallucinations aren’t a bug; they’re a mirror reflecting our own oversight.” Fixing them needs humility, rigour, and a dedication to the truth.
Are you intrigued by the possibilities of AI? Let’s chat! We’d love to answer your questions and show you how AI can transform your industry. Contact Us